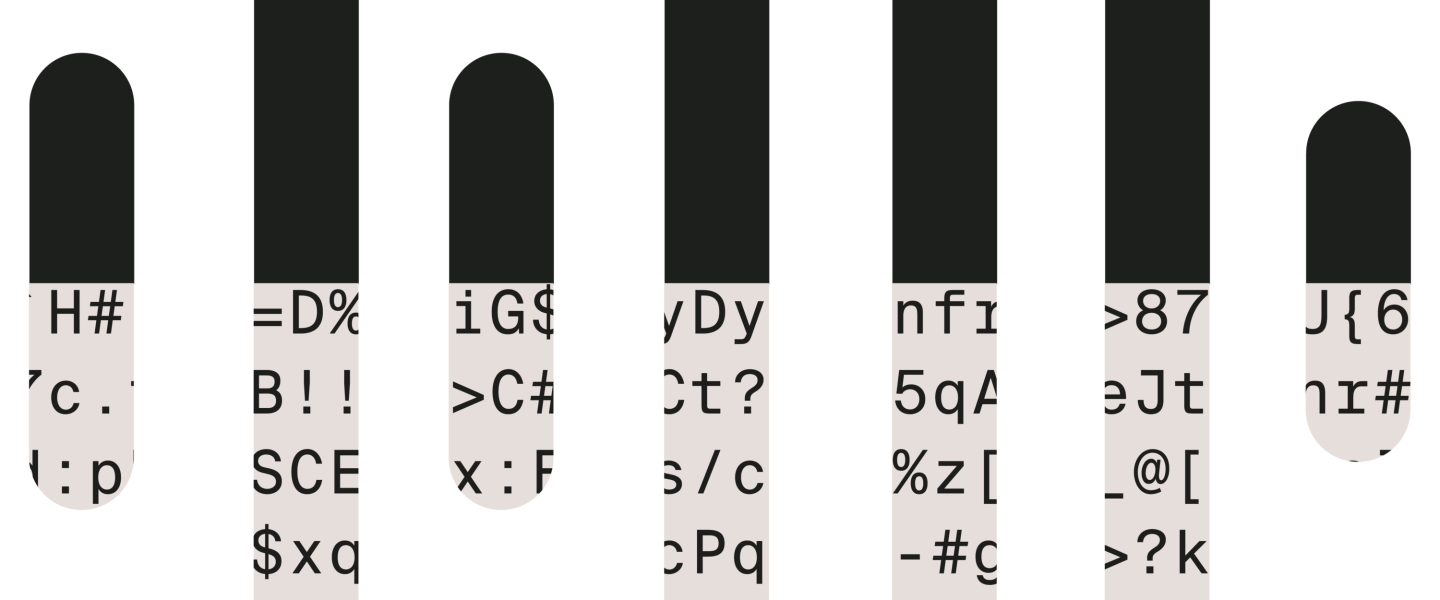
How can AI deliver on its promises in healthcare?
In theory, AI has much to offer healthcare, but developing successful applications in recent years has proved more difficult than thought. Most AI solutions are still in the experimental pre-clinical stage. Three UvA researchers highlight how the gap between science and medical practice can be closed.
'It’s just completely obvious that in five years deep learning is going to do better than radiologists.'
So said AI pioneer Geoffrey Hinton in 2016. He even claimed that training radiologists would no longer be necessary. Anno 2024, we can conclude that the implementation of AI in medical imaging has proven much more difficult than Hinton thought. No radiologist has become obsolete; rather, there is a shortage of radiologists.
Hinton won the 2018 Turing Award (the highest scientific award in computer science), along with Yann LeCun and Yoshua Bengio, for developing deep learning (a method by which computers learn to recognize patterns in data thanks to artificial neural networks). Hinton may know all about deep learning, but he knows little about the medical field, and that lack of domain knowledge broke him down in his prediction.
Imperfect labels
'I think it was embarrassing for Hinton to make such a strong claim', says associate professor Hoel Kervadec, a medical imaging researcher in the Quantitative Healthcare Analysis (qurAI) group at the UvA. 'We are far from being there, and actually replacing radiologists is not the goal at all.'
The large gap between Hinton’s 2016 statement and the reality in 2024 illustrates a deeper underlying problem, Kervadec explains. 'AI researchers often think of radiological images as a collection of perfect data, but there is much more variation and uncertainty in the data than they realize. The biggest problem is that the labels radiologists assign to a piece of an image are much more subjective than is often thought. What is tumor tissue and what is not? What type of tumor is involved? Radiologists can differ in their opinions on this.'
In addition, there is a large imbalance between the number of scans of healthy people and the number of scans of sick people, something standard AI methods struggle to deal with. Also, the fact that different types of scanners and different scanning protocols are used affects the conclusions an AI system draws, when it really shouldn’t matter.
'Instead of thinking that AI replaces radiologists, you can also say that AI ensures that the same number of radiologists can review more scans and better meet the high demand.'
Grand challenges
Applying AI techniques reliably in the complex reality of the hospital requires a lot of work. One way to narrow the gap between AI in the lab and AI the hospital is to organize Grand Challenges, such as the Artificial Intelligence for RObust Glaucoma Screening challenge (AIROGS) organized by some UvA colleagues of Kervadec in 2022. The goal of AIROGS was to detect the eye disease glaucoma at an early stage. It turned out that the best teams participating in this Grand Challenge performed similarly to an expert team of ophthalmologists and optometrists.
'Looking at the impressive results of such challenges in recent years', says Kervadec, 'I expect AI to slowly creep into hospital practice.' Crucial to this is close collaboration between the developers of the AI systems and the radiologists who want to work with them. They have the necessary domain knowledge that the AI researchers themselves do not have. Kervadec: 'We will have to learn what works well and what doesn’t.'
Kervadec himself specializes in developing new methods of medical imaging that deal more efficiently with big data. 'I try to use knowledge that doctors already have beforehand to let AI perform a certain task faster and better. For example, think of a doctor using certain anatomical knowledge to draw the contour around a certain piece of tissue.'
Kervadec sees the introduction of AI in medical imaging not as a replacement for human radiologists, but as a more than welcome addition. 'People now often have to wait a long time for a result. And in many Western countries the population is aging, increasing the demand for radiological screening. In other countries, there are not enough radiologists at all. So instead of thinking that AI replaces radiologists, you can also say that AI ensures that the same number of radiologists can review more scans and better meet the high demand.' This can be done, for example, by leaving the simple cases to AI.
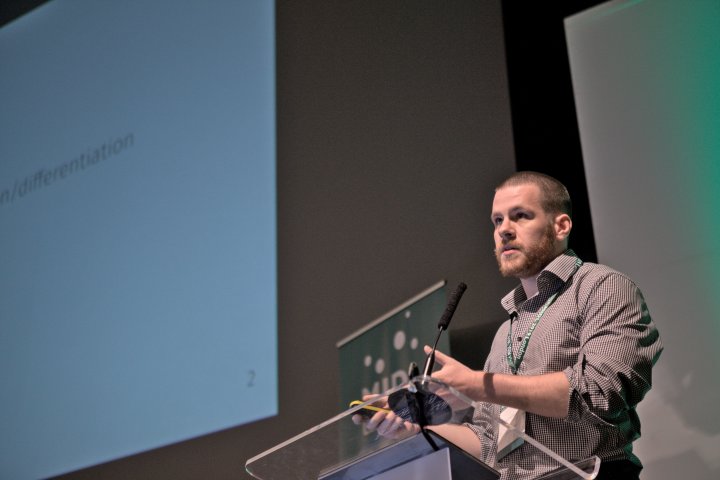
Medical startup
To bridge the gap between scientific research and medical practice, Evangelos Kanoulas, UvA professor of Information Retrieval and Evaluation, founded a startup company five years ago: Ellogon.ai. This startup aims to help medical experts select the right patients for cancer immunotherapy. Of all cancer patients who receive immunotherapy, only thirty percent currently benefit from it. But the cost is extremely high: about 250,000 euros per patient.
'The goal of Ellogon.ai,' Kanoulas explains, 'is to develop an AI tool that will allow doctors to better determine who does and does not benefit from immunotherapy.' In current practice, a histopathologist studies a piece of tumor tissue to determine whether or not a patient will receive immunotherapy. One important condition is that the area around the tumor must contain at least thirty percent immune cells. Another condition is that tumor cells must have less than a certain amount of blocking protein in their cell membrane, otherwise immune cells have no chance of eliminating the tumor cells.
'In Europe, very few investors are willing to invest in healthcare, because they find it too slow to pay off. That leads to companies leaving for the U.S. at some point. Europe needs to do a better job of creating an attractive environment for healthcare startup companies. Surely it is more useful to improve healthcare with AI, than to develop the next Angry Birds game.'
'Quantifying these kinds of biomarkers is difficult even for well-trained histopathologists,' Kanoulas says. 'It is actually a task for which the human eye is not suited. We see this in the fact that only three out of ten histopathologists agree with each other when looking at the same piece of tumor tissue. The AI software we develop to quantify biomarkers does not get tired, provides consistent quality and performs slightly better than the best human experts. And that’s certainly better than a random expert in a random hospital.'
But it’s not necessary that AI performs better. Even when AI does as well as human experts, it solves a problem. 'After all, there is a shortage of histopathologists', Kanoulas says. 'So it would help tremendously when our AI software does the easy cases and the histopathologists focus on the difficult cases.'
Still, there are also unknown variables in tumor tissue that determine whether or not a patient will benefit from immunotherapy. Kanoulas hopes that Ellogon.ai’s software can put human experts on track to unravel those as well: 'AI can see patterns that the human eye cannot see.' The software now has an official CE mark, but is not yet used in clinical practice. Kanoulas and his team are working closely with the Netherlands Cancer Institute (NKI) to investigate whether patients will also benefit from the developed AI support in practice.
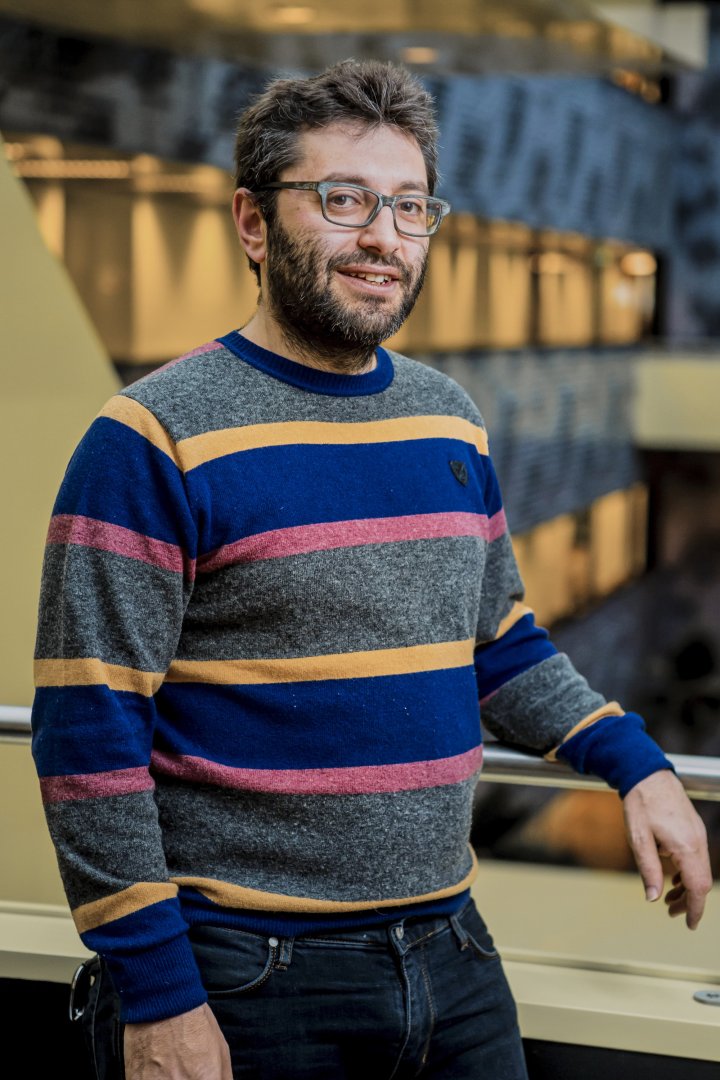
The complexity of AI in the hospital
As someone who stands with one leg in science and the other in practical application, how does Kanoulas view narrowing the gap between AI in the lab and AI in the hospital? What obstacles are there?
'First of all, healthcare is a complex, interdisciplinary field,' he says. 'In our field, AI experts and software engineers collaborate with histopathologists, oncologists and other medical professionals.'
In addition, health care involves people’s lives, so great care is required. New applications must therefore comply with laws and regulations. Kanoulas: 'Within the EU, these include the Medical Device Regulation (MDR), the In Vitro Diagnostic Medical Devices Regulation (IVDR), and when it comes to privacy and the use of digital data, the General Data Protection Regulation (GDPR) applies.'
[read more below the gallery] ⬇️
What does AI have to offer health care?
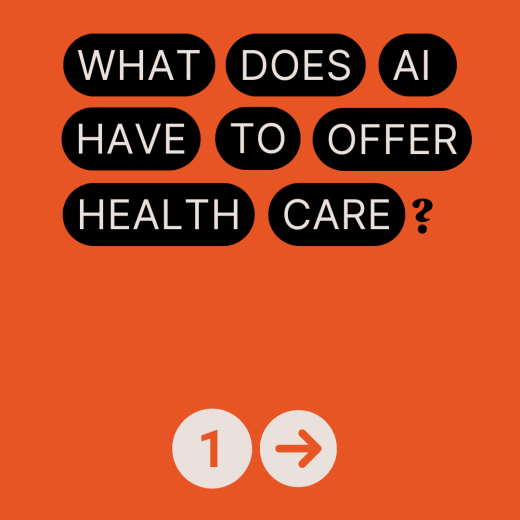
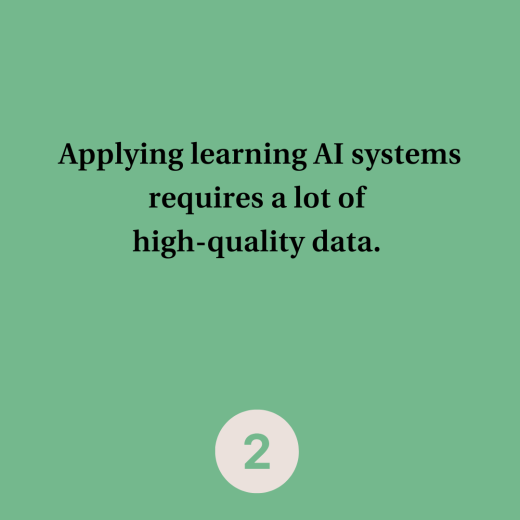
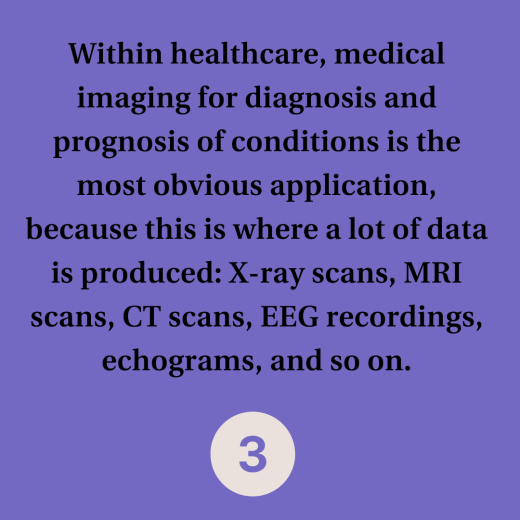
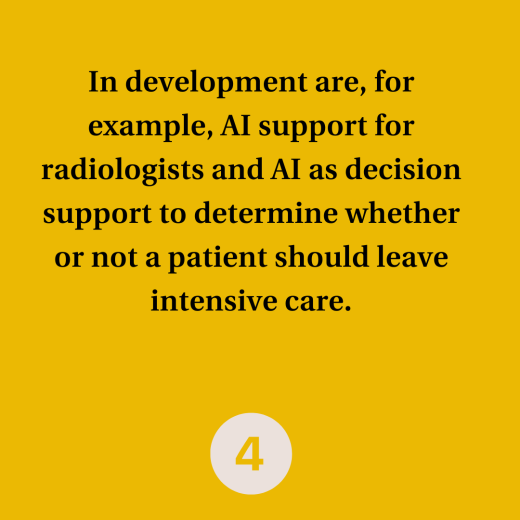
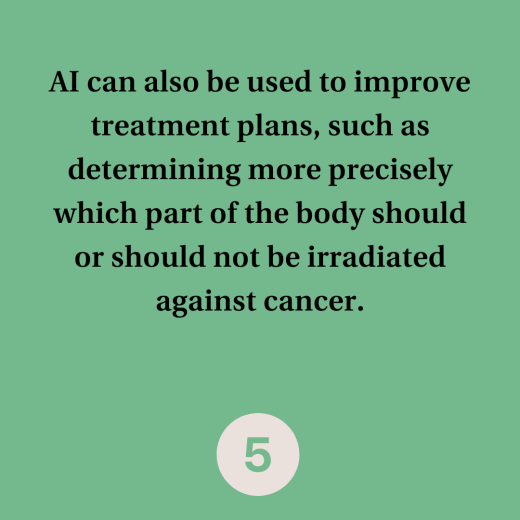
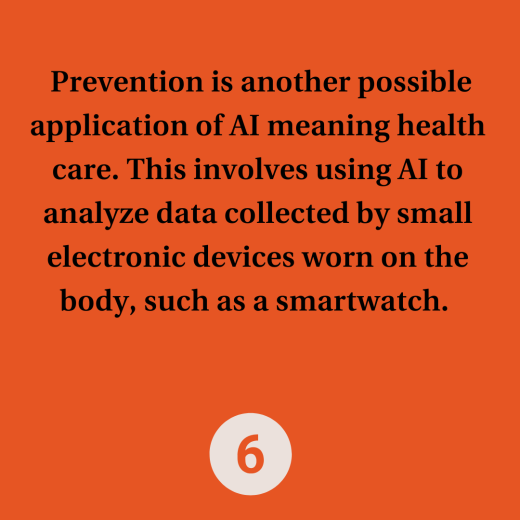
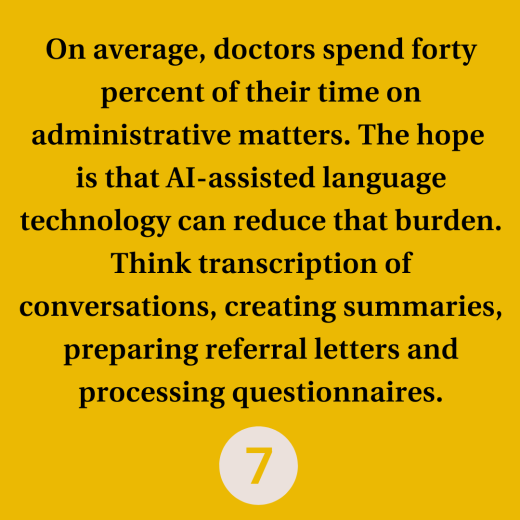
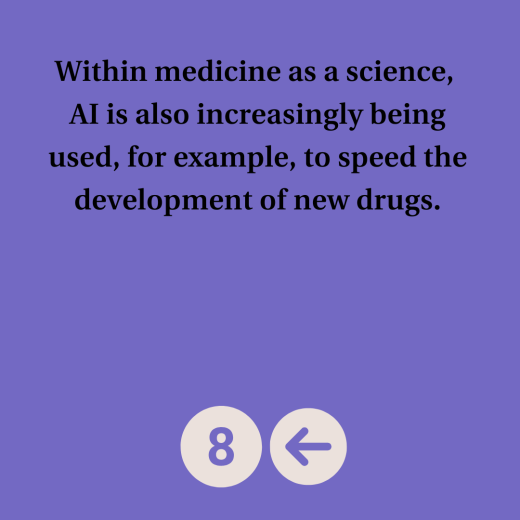
Third, healthcare has a long tradition that makes major changes difficult. Kanoulas: 'AI needs digital data. That means hospitals have to have a digitization strategy. That costs money and that changes the workflow.'
And finally, of course, there are financial barriers. Kanoulas: 'For the hospital now, by and large from a financial perspective, it doesn’t matter if a certain treatment that is in the health insurance package works or not. The health insurance company pays for it anyway. It takes a long time to get a new product reimbursed by health insurance. Many startup companies that have valuable products don’t make it for that reason.'
Another financial barrier is the money to invest in research and development at all as a startup. 'In Europe, very few investors are willing to invest in healthcare, because they find it too slow to pay off', says Kanoulas. 'That leads to companies leaving for the U.S. at some point, and frankly, that’s something we are also thinking about with Ellogon.ai. Europe needs to do a better job of creating an attractive environment for healthcare startup companies. I see that as socioeconomic value creation. Surely it is more useful to improve healthcare with AI than to develop the next Angry Birds game.'
'AI applications in diagnostics are important, but AI also lends itself well to improving steps much earlier in the care chain. Think of a chatbot that helps people with their healthcare questions or AI that can help people with early-stage dementia live independently longer by, for example, helping them remember when to eat or drink. That AI would then have to communicate in an empathetic way. These are some ideas we want to explore further in the coming years.'
Equal access to healthcare
Somaya Ben Allouch, professor of Human-System Interaction for Health and Wellbeing at the UvA, is also working closely with the practical field to integrate AI applications into health care as well as possible. Ben Allouch does so primarily with the goal of understanding how to develop AI applications that fit within the workflow of clinicians and the care settings. Another important aim is reducing inequitable access to health care.
'It is known that some groups in society have worse access to healthcare than other groups', Ben Allouch says. 'This is related, for example, to ethnicity, low literacy or lower socioeconomic status. In some neighborhoods in big cities, people skip the general practitioner. If there is something wrong, they go straight to the hospital first-aid station. That may be because they work during the day, because a language barrier makes it inconvenient for them to have to talk to an assistant first, or because they just want to be helped faster.'
Ben Allouch is working with patient organizations, social assistance, GGD and the City of Amsterdam, among others, to explore how AI can help ensure, on the one hand, more equal access for all groups, and on the other hand, that if AI is used in health care, it is not accompanied by discrimination against certain groups. 'We are at the very beginning of our research', says Ben Allouch. 'But the idea is that from day one, we are proactively talking to all these stakeholders about what is needed for more equal access: What data do we need? How do we clean that data? What biases are at play?'
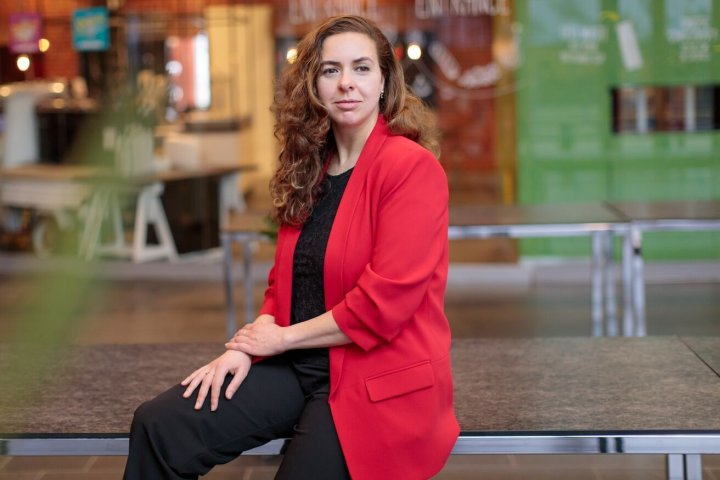
Ben Allouch’s research will be embedded in the AI for Health Equity Lab, a partnership of Amsterdam University of Applied Sciences (HvA), University of Amsterdam (UvA), Vrije Universiteit (VU), and Amsterdam UMC. Earlier this year, this lab was awarded the ELSA (Ethical, Legal and Societal Aspects) label by the Dutch AI Coalition (NL AIC).
'Of course AI applications in diagnostics are important,' says Ben Allouch, 'but let’s not be blinded by it. AI also lends itself well to improving steps much earlier in the care chain. Think of a chatbot that helps people with their healthcare questions without misunderstanding them all the time. Or think of AI that can help people with early stage dementia live independently longer by, for example, helping them remember when to eat or drink. That AI would then have to communicate in an empathetic way. These are some ideas we want to explore further in the coming years.'
Ben Allouch emphasizes that we should not have blind faith in healthcare technology, whether AI or other digital healthcare solutions. 'I have done all kinds of technology projects to investigate how we can support the elderly. Then you see that the meaningful, human relationship between a person in need of care and a care professional, a child, a parent or a neighbor cannot just be replaced by a piece of technology. I am in favor of AI applications, but let’s critically examine and reflect upon what is and is not needed and what does or does not work in practice and its effect on humans.'
About the interviewees
- Somaya Ben Allouch
Professor by special appointment of Human-System Interaction for Health and Wellbeing. - Evangelos Kanoulas
Professor of Information Retrieval and Evaluation. Leading the Human-Machine Intelligence Lab (HuMIL), within the Informatics Institute and the ILPS group. Co-founder of and Chief Operation Officer at Ellogon.AI - Hoel Kervadec
Associate professor and Medical Imaging researcher in the Quantitative Healthcare Analysis (qurAI) group.