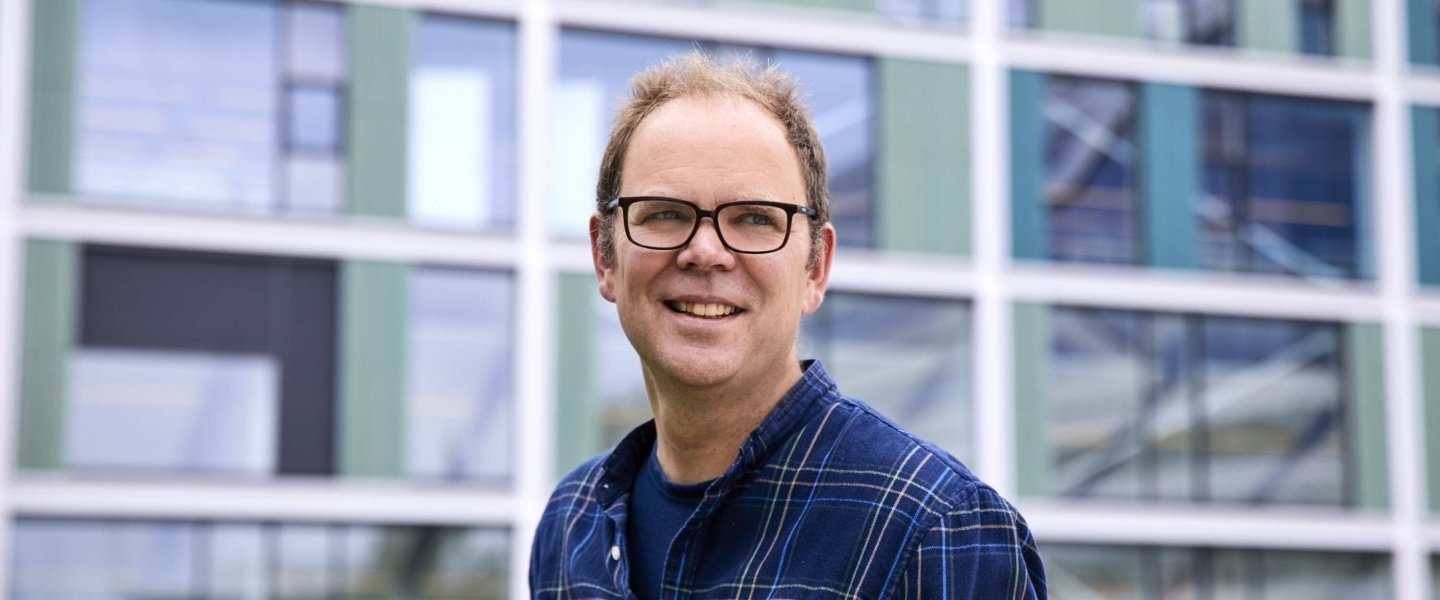
Jelle Zuidema: Finding out why AI makes certain choices
It's a well-known example: in 2015, it became painfully clear that the application of AI can sometimes go terribly wrong. A month previously, Google had introduced the option of automatically tagging photos and putting them in an album. Very convenient in itself, you might say. However, the system got it very wrong in the case of a photo of a dark-skinned man and his girlfriend. Their photo was tagged ‘gorilla’. The app received a lot of criticism, and Google promised to remedy the error. But guess what? Three years later, the error had not been corrected at all; it turned out that Google had simply blocked the ‘gorilla’ category.
The fact that Google is unable to resolve this issue indicates the kinds of problems we are facing within AI. ‘The algorithms we are using have become extremely good, but at the same time so complex that these kinds of sexist or racial prejudices are not easy to eliminate', says Jelle Zuidema, associate professor of Computational Linguistics & Explainable AI.
‘Computers have become self-learning through deep learning. As a result, we don’t know exactly which rules they are using. This is the black box we are facing.’
Research into the black box
Basically, what it comes down to is that scientists don't really know how the computer comes up with a certain answer. ‘Computers have become self-learning through deep learning. As a result, we don’t know exactly which rules they are using. This is the black box we are facing.’ And this black box is precisely what Zuidema is studying. ‘Researching this black box is actually a new science that translates the values in the mass of numbers that computers work with into descriptions that can be interpreted by people.’
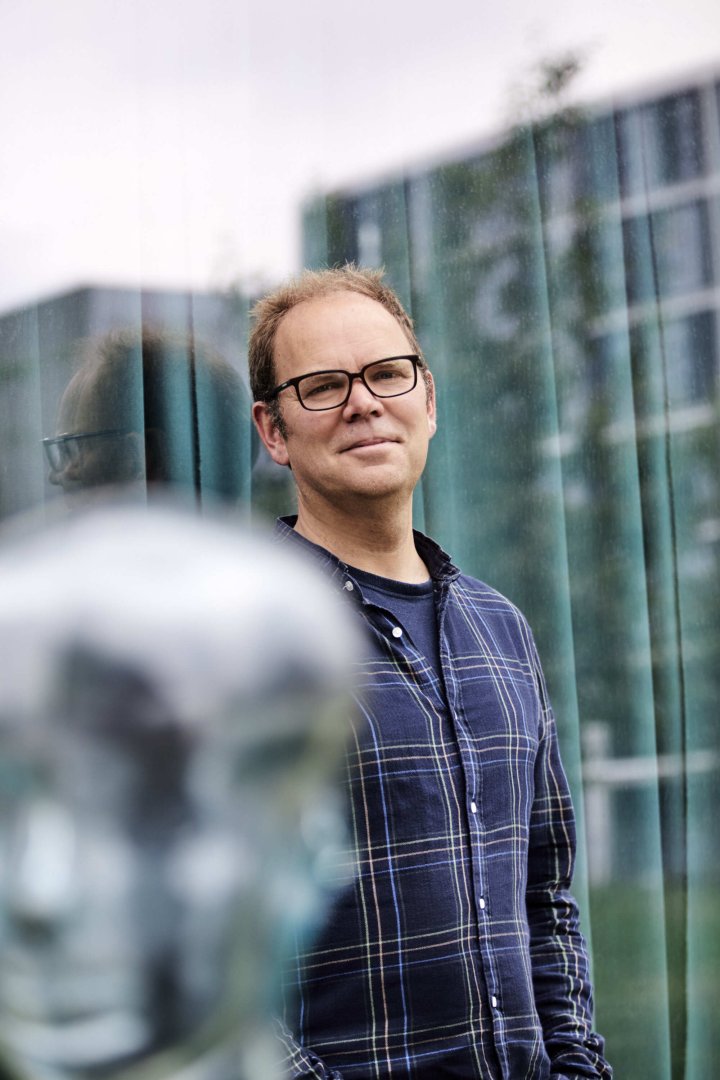
But how can it be that scientists no longer understand the decisions of machines they themselves developed? Jelle: ‘You could compare it with the development of medicine. Although there are a lot of steps in this process that we do understand, in some cases we still don't know why molecule X has an effect on disease Y. We have now also reached this stage with AI. We know that AI comes up with certain answers, but not exactly why. We still need to do a lot more research in this area.’
We form hypotheses and then test them. For example: in order to distinguish a mouse from a dog, a deep learning system must at least represent the shape of the ears. Or the whiskers. Then, you can explicitly search for the "are there whiskers here?" detector.
Looking for whiskers
In his research, Jelle uses specific studies in the areas of neuroscience and psychology as an example. ‘These are disciplines that have been researching the opening of a black box, namely the human brain, for hundreds of years now. When studying the visual perception of the brain, for example, you have an activity known as probing. By measuring a certain activity in the brain cells, you can see which animal your brain has observed.'
'We do the same thing with AI. We form hypotheses and then test them. For example: in order to distinguish a mouse from a dog, a deep learning system must at least represent the shape of the ears. Or the whiskers. Then, you can explicitly search for the "are there whiskers here?" detector.'
Finding out why AI makes certain choices
Not knowing why an algorithm does something can be very problematic, for example in the case of medical diagnoses. Jelle: ‘Take the early detection of cancer. Radiologists can use AI to detect this, but you can get into a situation in which the computer correctly indicates the presence of cancer, but can’t explain on which basis it reached this conclusion. Let alone exactly where the cancer is located. And if you don't know where the incipient cancer is, it means you also won't be able to remove it.’
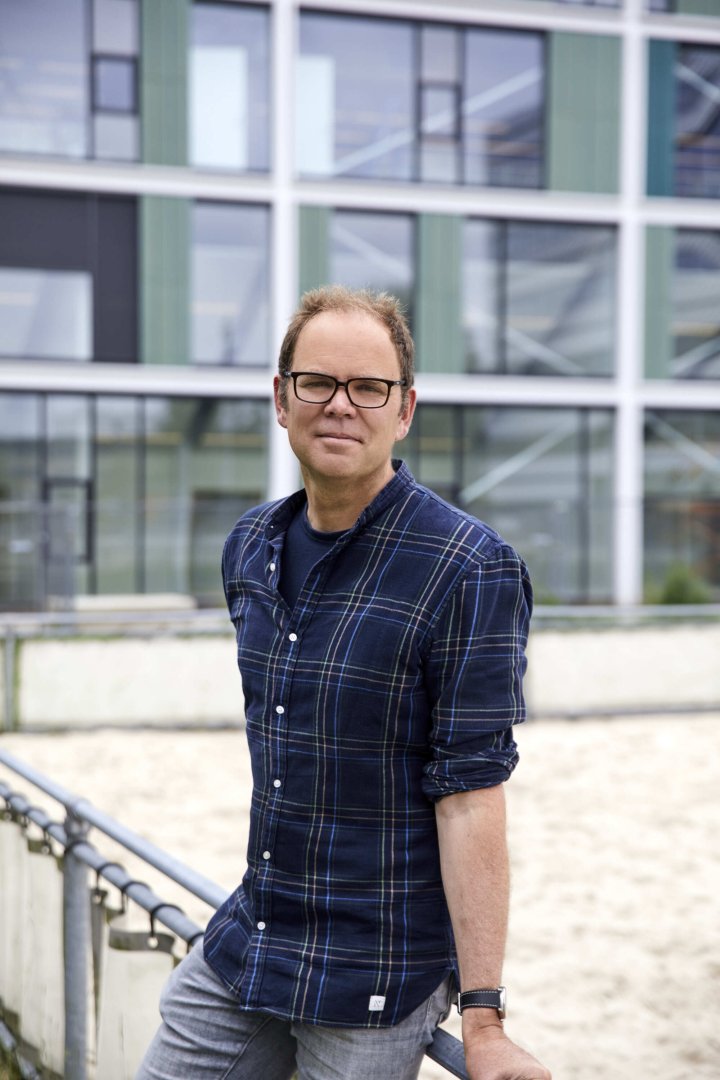
He gives another example. ‘Spotify also uses Deep Learning. Often, we don't know why the search algorithm recommends certain songs. Users often find this very frustrating. They think: ‘‘I just put on some nice Indie Rock, so why am I suddenly hearing classical music?” They also want more control, but in order to give them this control, as scientists we must first have more control over "why" AI makes certain choices.’
Working in LAB42
‘The Institute for Logic, Language and Computation, to which I am attached, has always had a considerable ivory tower culture that focuses on top science worldwide, but pays less attention to applications. And that’s a shame, because some of the things we focus on don’t really differ all that much from the applied AI problems people are facing in the business world. For example, take the question: ‘How can we ensure that our machine translation tool is not gender biased?’ I hope that LAB42 will help to break through this ivory-tower culture a little.’
About the series Meet the residents
LAB42 comes alive! This new international AI and digital innovation hub at Amsterdam Science Park will be opened in September 2022. The aim is to build a community that fosters stronger connections between companies, students and researchers. LAB42 is a unique, international place for knowledge and talent development.
In this series, we explore the motives and drivers of the future residents of the building.
About Jelle Zuidema
- Working as an associate professor in computational linguistics and cognitive science at the Institute for Logic, Language and Computation.
- More information on Jelle’s personal page on the UvA site.