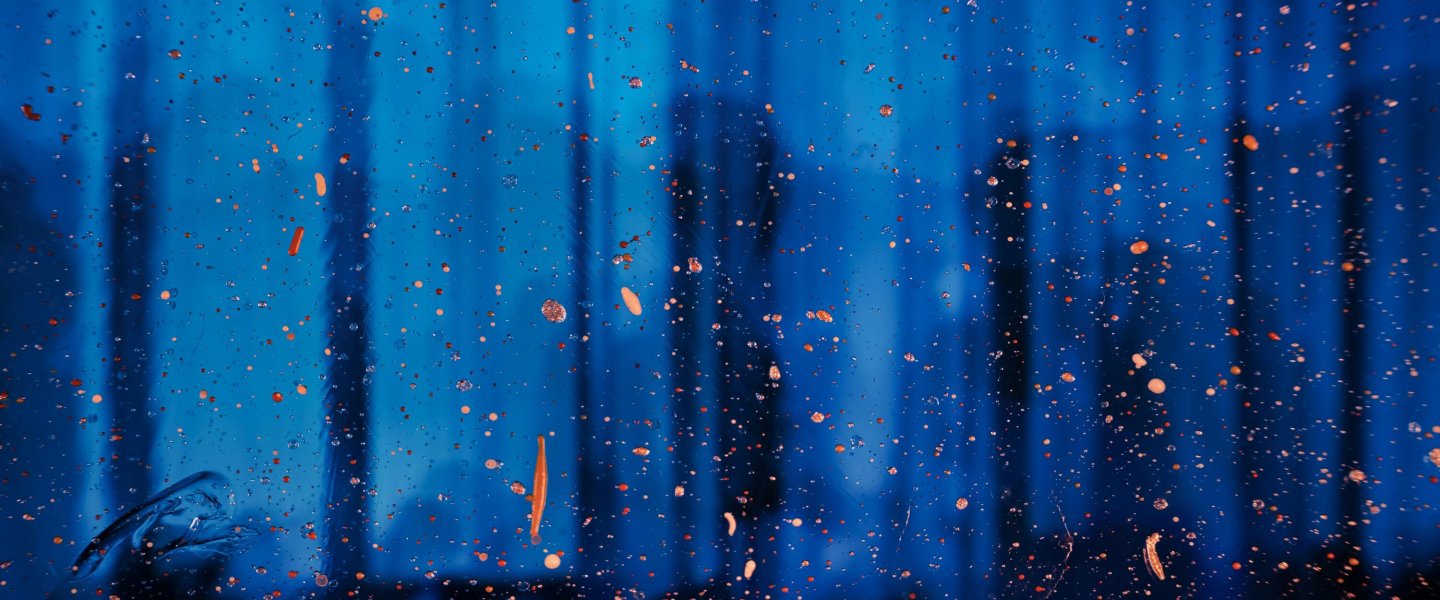
LAB42 Talk | SEA: Explainable AI
In this edition of SEA, we will discuss explainability in AI with two great speakers: Meike Nauta (Datacation, Universiteit Twente) and Lijun Lyu (TU Delft). This is a hybrid event. The in-person event takes place at Lab42, Science Park, room L3.36.
IMPORTANT: You can view the Zoom link once you have 'attended' the meetup on the meetup page.
Speaker: Meike Nauta (Datacation, Universiteit Twente)
Title: Power to the people with the power of AI
Time: 17:00
Abstract: Meike Nauta will give an overview of explainable AI methods and how to evaluate them while showing both the possibilities and risks of using them. She will then present her view on the future of explainable and responsible AI: interpretability-by-design as an alternative for the black box, in line with her vision for responsible AI: power to the people with the power of AI.
SEA Talk #264
Speaker: Lijun Lyu (TU Delft)
Title: Is Interpretable Machine Learning Effective at Feature Selection for Neural Learning-to-Rank?
Abstract: Neural ranking models have become increasingly popular for real-world search and recommendation systems in recent years. Unlike their tree-based counterparts, neural models are much less interpretable. That is, it is very difficult to understand their inner workings and answer questions like how do they make their ranking decisions? or what document features do they find important? This is particularly disadvantageous since interpretability is highly important for real-world systems. In this work, we explore feature selection for neural learning-to-rank (LTR). In particular, we investigate six widely-used methods from the field of interpretable machine learning (ML) and introduce our own modification, to select the input features that are most important to the ranking behavior. To understand whether these methods are useful for practitioners, we further study whether they contribute to efficiency enhancement. Our experimental results reveal a large feature redundancy in several LTR benchmarks: the local selection method TabNet can achieve optimal ranking performance with less than 10 features; the global methods, particularly our G-L2x, require slightly more selected features, but exhibit higher potential in improving efficiency. We hope that our analysis of these feature selection methods will bring the fields of interpretable ML and LTR closer together.
SEA Talk #265